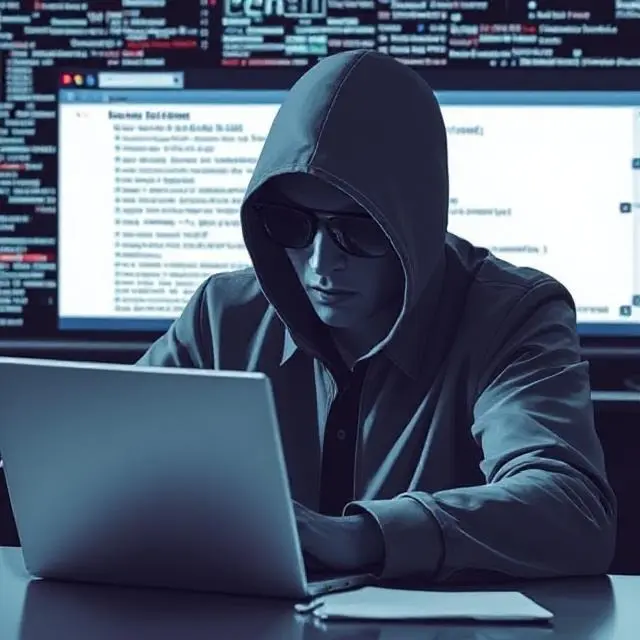
In today's digital era, online fraud has become one of the most concerning issues for individuals and businesses alike. With the increasing number of transactions conducted online, fraud has also become more sophisticated and difficult to detect. However, with advancements in technology, particularly in the field of artificial intelligence (AI), online fraud detection has become more effective and efficient. This article will discuss how AI assists in detecting online fraud, the techniques used, and the benefits it provides to users and companies.
1. What is Online Fraud?
Online fraud refers to various fraudulent activities conducted over the internet, including identity theft, credit card fraud, and phishing schemes. Such fraud can lead to significant financial losses for individuals and businesses. Therefore, it is crucial to have an effective system in place to detect and prevent these fraudulent activities.
2. The Role of Artificial Intelligence in Fraud Detection
Artificial intelligence plays a vital role in detecting online fraud by analyzing vast amounts of data and identifying suspicious patterns. AI can process information quickly and accurately, allowing for real-time fraud detection. Here are some ways AI is utilized in fraud detection:
Big Data Analysis: AI can analyze large volumes of transaction data to find unusual patterns. For instance, if someone makes a large transaction from an unusual location, the AI system can flag that transaction as suspicious.
Machine Learning: Machine learning algorithms can be trained to recognize fraud patterns based on historical data. By using this technique, the system can learn from past transactions and improve the accuracy of fraud detection.
Anomaly Detection: AI can identify anomalies in data that may indicate fraud. For example, if there is a sudden spike in the number of transactions from a single account, the system can alert the security team.
3. Techniques Used in Fraud Detection
Several techniques are employed by AI in detecting online fraud:
Logistic Regression: This technique is used to predict the likelihood of fraud occurring based on existing variables. By using historical data, a logistic regression model can provide the probability that a transaction is fraudulent.
Decision Trees: This method divides data into branches based on specific criteria. Each branch represents a decision made based on the analyzed data. This helps in identifying suspicious transactions.
Neural Networks: Neural networks can be used to recognize complex patterns in data. By training the neural network on transaction data, the system can learn to differentiate between legitimate and suspicious transactions.
Classification Algorithms: These algorithms are used to classify transactions into different categories, such as legitimate or suspicious. Examples of classification algorithms include Support Vector Machines (SVM) and Random Forest.
4. Benefits of Using AI in Fraud Detection
The use of AI in online fraud detection offers numerous benefits, including:
Speed and Efficiency: AI can process data in real-time, allowing for faster fraud detection compared to manual methods.
Higher Accuracy: By utilizing machine learning algorithms, the system can improve the accuracy of fraud detection over time, reducing the number of false positives (transactions flagged as fraud when they are legitimate).
Reduction of Financial Losses: By detecting fraud earlier, companies can minimize financial losses caused by fraudulent activities.
Increased Customer Trust: With an effective fraud detection system, customers will feel safer when conducting online transactions, enhancing their trust in the company.
5. Challenges in Fraud Detection Using AI
Despite the many benefits AI offers in fraud detection, there are several challenges that need to be addressed:
Imbalanced Data: In many cases, the number of legitimate transactions far exceeds the number of suspicious transactions. This can make it difficult for AI models to learn from imbalanced data.
Evolving Fraud Tactics: Fraudsters continuously develop new tactics to evade detection. Therefore, AI systems need to be updated regularly to remain effective.
Data Privacy: The use of personal data to train AI models can raise privacy concerns. Companies must ensure they comply with data privacy regulations when using AI for fraud detection.
6. Case Studies of AI in Fraud Detection
Many companies have successfully implemented AI in fraud detection. Here are some examples:
Banking: Many banks use AI to monitor transactions in real-time and detect suspicious activities. If the system detects an unusual transaction, the bank can immediately contact the customer to verify the transaction.
E-commerce: E-commerce platforms use AI to analyze purchasing patterns and detect fraud in transactions. If a suspicious transaction is identified, the system can suspend the transaction until further verification.
Insurance: Insurance companies use AI to analyze claims and detect suspicious claims. By employing machine learning algorithms, they can identify potentially fraudulent claims and conduct further investigations.
7. The Future of Fraud Detection with AI
With ongoing technological advancements, the future of fraud detection with AI looks promising. Some trends that may emerge in the future include:
Integration with Other Technologies: AI will increasingly integrate with other technologies, such as blockchain and the Internet of Things (IoT), to enhance security and fraud detection.
Use of Predictive Analytics: By employing predictive analytics, companies can anticipate potential fraud before it occurs, allowing for better preventive measures.
Enhanced User Experience: With improved fraud detection systems, the user experience in online transactions will become safer and more convenient.