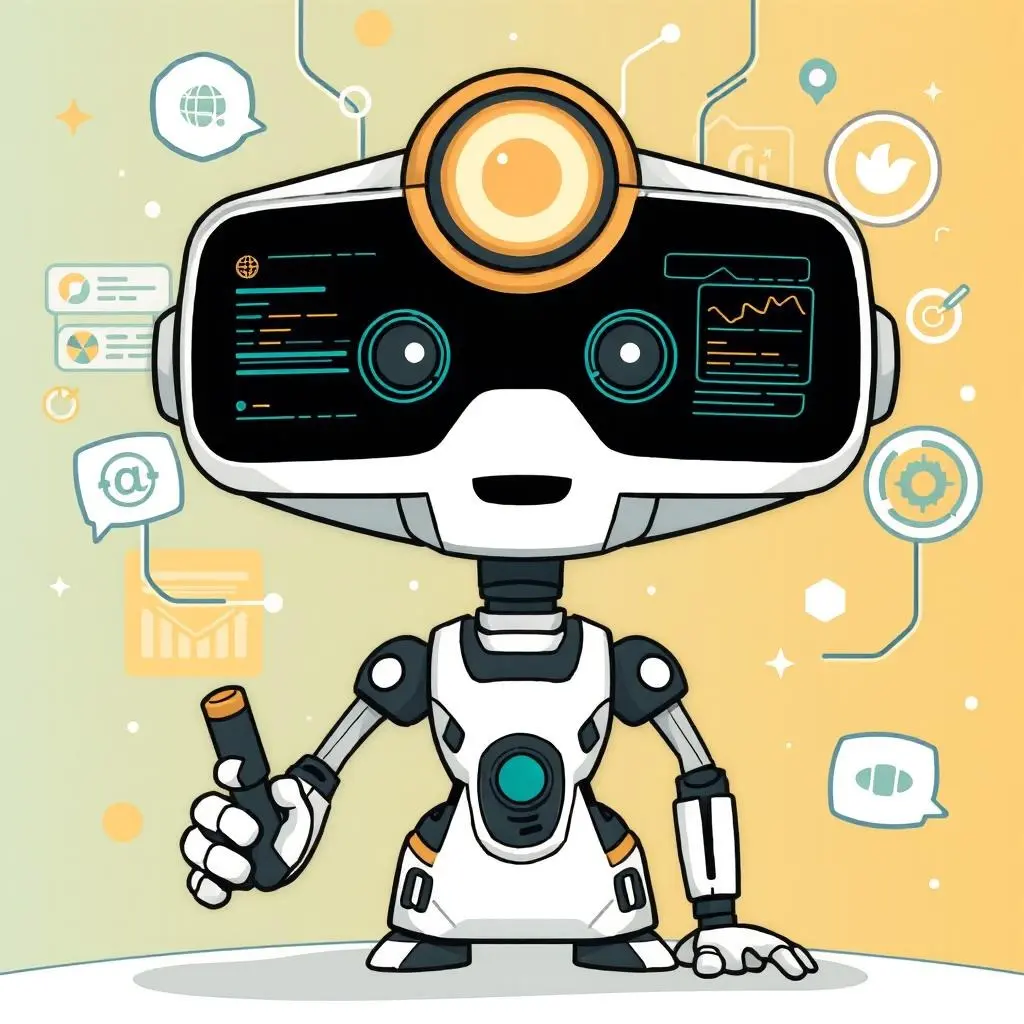
In today's digital age, data has become a valuable asset for every business. Data-driven marketing has become an essential approach to understanding customers, optimizing campaigns, and increasing ROI (Return on Investment). However, with the advancement of AI (Artificial Intelligence) and predictive analytics, data-driven marketing has reached a new level, becoming smarter, more efficient, and more personalized.
What is Data-Driven Marketing with AI and Predictive Analytics?
Conventional data-driven marketing focuses on collecting and analyzing historical data to understand past trends and campaign performance. Meanwhile, with AI and predictive analytics, we can go beyond descriptive analysis and move to predictive and prescriptive analysis. This means we not only understand what happened, but also why it happened and what might happen in the future.
AI and predictive analytics allow us to:
Automate Data Collection and Analysis: AI can automatically collect data from various sources, clean it, and analyze it quickly and accurately.
Predict Consumer Behavior: By analyzing data patterns, AI can predict future consumer behavior, such as purchasing preferences, churn probability, and responses to specific campaigns.
Personalize Marketing Campaigns: Based on predictions of consumer behavior, we can personalize marketing messages, offers, and customer experiences in real-time.
Optimize ROI: By identifying the most effective campaigns and allocating resources appropriately, we can maximize ROI.
Benefits of Data-Driven Marketing with AI and Predictive Analytics:
More Accurate Targeting: By deeply understanding customer profiles and preferences, we can target marketing campaigns to the right audience with relevant messages.
Smarter Decision Making: The data and insights generated by AI and predictive analytics provide a strong foundation for more strategic marketing decision-making.
Improved Customer Experience: AI-powered personalization creates a more relevant, engaging, and satisfying customer experience.
Increased Efficiency and Productivity: Automating data collection and analysis processes frees up marketing team time and resources to focus on more strategic tasks.
Examples of Implementation:
Product Recommendations: E-commerce platforms use AI to recommend products to customers based on their purchase history, browsing, and preferences.
Customer Segmentation: AI can group customers into segments based on their behavior and characteristics, enabling more targeted campaigns.
Churn Prediction: Telecommunications companies use predictive analytics to identify customers who are likely to switch to competitors and take appropriate retention measures.
Challenges and Considerations:
Data Quality: Poor data quality can lead to inaccurate predictions. It is important to ensure that the collected data is clean, complete, and relevant.
Data Privacy: The use of customer data must be done responsibly and in accordance with applicable data privacy regulations.
Technology and Resource Investment: Implementing AI and predictive analytics requires investment in technology and capable human resources.